Logistics is a complex process that involves managing the flow of goods and services from one location to another. It is essential to ensure that goods are delivered on time, and at the right place and cost. In recent years, logistics companies have started to use predictive analytics to plan and forecast their operations, and it has been a game-changer.
What is Predictive Analytics?
Predictive analytics is the use of data, statistical algorithms, and machine learning techniques to identify the likelihood of future outcomes based on historical data. In logistics, this means analyzing data on shipping routes, inventory levels, weather patterns, and other factors to predict demand, optimize routes, and reduce costs.
The Role of Predictive Analytics in Logistics Planning
Planning is the backbone of logistics. It involves coordinating resources, such as trucks, warehouses, and personnel, to ensure that goods are delivered on time. Predictive analytics can help logistics companies plan more efficiently by analyzing data on past shipments, inventory levels, and demand patterns to identify trends and make accurate predictions about future demand.
For example, if a logistics company knows that a particular product is popular during a specific time of year, they can adjust their operations to ensure that they have enough inventory on hand to meet the demand. By using predictive analytics, logistics companies can make data-driven decisions that optimize their operations and reduce costs.
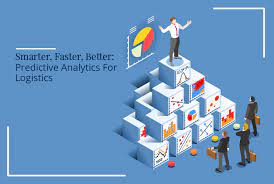
The Role of Predictive Analytics in Forecasting
Forecasting is another critical aspect of logistics. It involves predicting demand, inventory levels, and other factors that affect the flow of goods. Accurate forecasting is essential to ensure that logistics companies have enough inventory on hand to meet demand and avoid stockouts.
Predictive analytics can help logistics companies forecast more accurately by analyzing historical data and identifying trends. By using machine learning algorithms, logistics companies can make predictions about future demand based on historical patterns and other factors, such as weather and seasonal trends.
For example, a logistics company can use predictive analytics to identify patterns in demand for a particular product. They can then use this data to adjust their inventory levels and plan their operations accordingly, reducing the risk of stockouts and ensuring that they have enough inventory on hand to meet demand.
The Benefits of Predictive Analytics in Logistics Planning and Forecasting
The use of predictive analytics in logistics planning and forecasting offers several benefits. These include:
- Improved Efficiency: By using predictive analytics, logistics companies can optimize their operations, reducing costs and improving efficiency.
- Better Inventory Management: Predictive analytics can help logistics companies manage their inventory more effectively, reducing the risk of stockouts and ensuring that they have enough inventory on hand to meet demand.
- Improved Customer Service: By using predictive analytics, logistics companies can ensure that they deliver goods on time, improving customer satisfaction and loyalty.
- Competitive Advantage: Logistics companies that use predictive analytics have a competitive advantage over those that don’t. They can make data-driven decisions that optimize their operations, reducing costs and improving efficiency.
Predictive analytics is revolutionizing logistics planning and forecasting. By analyzing data on past shipments, inventory levels, and demand patterns, logistics companies can make data-driven decisions that optimize their operations and reduce costs. The benefits of predictive analytics include improved efficiency, better inventory management, improved customer service, and a competitive advantage. As logistics companies continue to face increasing pressure to improve efficiency and reduce costs, predictive analytics will become an essential tool for success.
Predictive analytics can help logistics companies manage their operations more effectively by providing real-time insights into inventory levels, shipping routes, and other key performance indicators. With this information, logistics companies can quickly adapt to changes in demand, weather patterns, and other factors that affect the flow of goods.
For example, if a logistics company experiences a sudden increase in demand for a particular product, they can use predictive analytics to identify alternative shipping routes or adjust their inventory levels to meet the demand. This ability to quickly adapt to changes in the market is essential for logistics companies that operate in a rapidly changing environment.
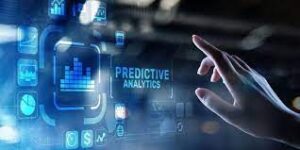
In addition to improving efficiency and reducing costs, predictive analytics can also help logistics companies improve their customer service. By providing real-time information on shipping times and inventory levels, logistics companies can keep their customers informed and ensure that they receive their goods on time. This improves customer satisfaction and loyalty, which is critical in an industry where competition is fierce.
One area where predictive analytics is particularly useful is in supply chain management. Supply chain management involves managing the flow of goods and services from suppliers to customers. It is a complex process that involves multiple parties, including manufacturers, distributors, retailers, and logistics companies. Predictive analytics can help these parties collaborate more effectively by providing real-time insights into inventory levels, shipping times, and other key performance indicators.
For example, a manufacturer can use predictive analytics to identify trends in demand for their products. They can then use this information to adjust their production schedules and ensure that they have enough inventory on hand to meet demand. A distributor can use predictive analytics to identify the most efficient shipping routes and reduce transportation costs. A retailer can use predictive analytics to identify trends in customer behaviour and adjust their marketing strategies accordingly.
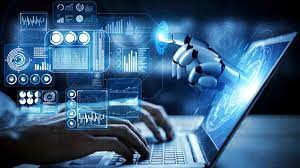
Another area where predictive analytics is useful is in risk management. The logistics industry is subject to a variety of risks, including weather events, supply chain disruptions, and security threats. Predictive analytics can help logistics companies identify potential risks and take proactive steps to mitigate them.
For example, a logistics company can use predictive analytics to identify areas of their supply chain that are most vulnerable to disruption. They can then take steps to diversify their supply chain or invest in backup systems to ensure that they can continue to operate in the event of a disruption.
Conclusion
The role of predictive analytics in logistics planning and forecasting is becoming increasingly important. By providing real-time insights into inventory levels, shipping routes, and other key performance indicators, predictive analytics can help logistics companies improve efficiency, reduce costs, and improve customer service. As the logistics industry continues to evolve and become more complex, the use of predictive analytics will become an essential tool for success.